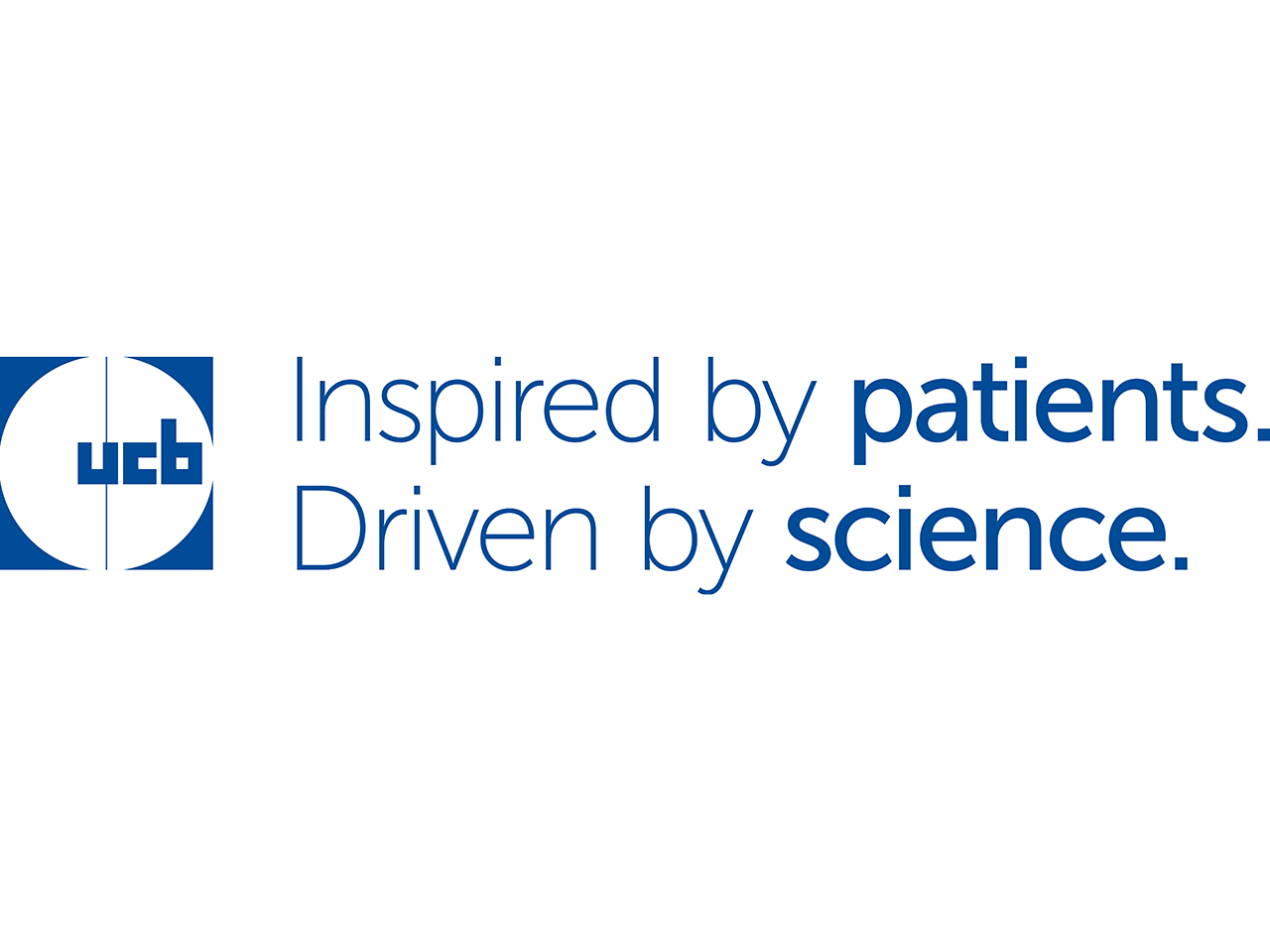
This weekend at the 2018 American Epilepsy Society Meeting (AES) in New Orleans, Louisiana we are highlighting UCB’s commitment to delivering patient value through innovative solutions designed to help ensure the right treatments and care reach patients living with severe diseases.
To advance this goal in epilepsy care, we are developing eliprio®, a suite of predictive analytics tools and capabilities which marries our clinical expertise in epilepsy with big data and computational science. These solutions aim to help identify patients who are at high risk of failing through multiple AEDs and to support treatment selection. Several abstracts on our work to date are being presented.
Driving our approach to eliprio®’s development is our understanding of the needs of epilepsy patients and their journey. In a global study of epilepsy patients, of the 70% who are now well managed, most report it took an average 2 to 3 years to get to that point and worry about repeating the process if anything were to change.1
The eliprio® solutions under development are predictive algorithms based on a data set that includes de-identified information on millions of patients over 10 years. One of the solutions currently in development is a drug-resistant epilepsy prediction application. The application is designed to help identify patients at high risk of developing drug resistant epilepsy (defined in the application as failing three or more distinct AEDs). This may signal when to refer a patient to a specialist or consider alternative treatment paths, and could help get patients to more appropriate care faster. The methodology of the DRE model was recently published in the journal Epilepsy & Behavior.2
UCB remains committed to delivering what patients value. For those living with epilepsy, achieving seizure control fast is an important part of living their ideal. We will continue exploring and innovating to find solutions that help patients achieve that ideal.
Sources:
1 Data on file. HOPE Study. 2014-2015. Smyrna, GA: UCB, Inc.
2 An S, Malhotra K, Dilley C, et al. Predicting drug-resistant epilepsy-A machine learning approach based on
administrative claims data. Epilepsy Behav. 2018;89:118-125.
Choose Country
- Global Site – English
- Australia – English
- België – Engels
- Belgique – Anglais
- Brasil – Português
- България – Български
- Canada – English
- Canada – Français
- 中国 – 中文
- Česká Republika – Angličtina
- Danmark – Engelsk
- Deutschland – Deutsch
- France – Français
- España – Español
- Ελλάδα – Ελληνικά
- India – English
- Ireland – English
- Italia – Inglese
- 日本 – 日本語
- Казахстан – ағылшын тілі
- 한국 – 한국어
- Luxembourg – Anglais
- Luxemburg – Engels
- Magyarország – Angol
- México & Latinoamérica – Español
- Nederland – Engels
- New Zeeland – English
- Norge – Engelsk
- Österreich – Deutsch
- Polska – Polski
- Portugal – Inglês
- România – Engleză
- Россия – Русский
- Slovensko – Anglický
- Suomi – Englanti
- Sverige – Engelska
- Schweiz – Deutsch
- Suisse – Français
- Türkiye – Türkçe
- Україна – Англійська
- United Kingdom – English
- U.S.A. – English